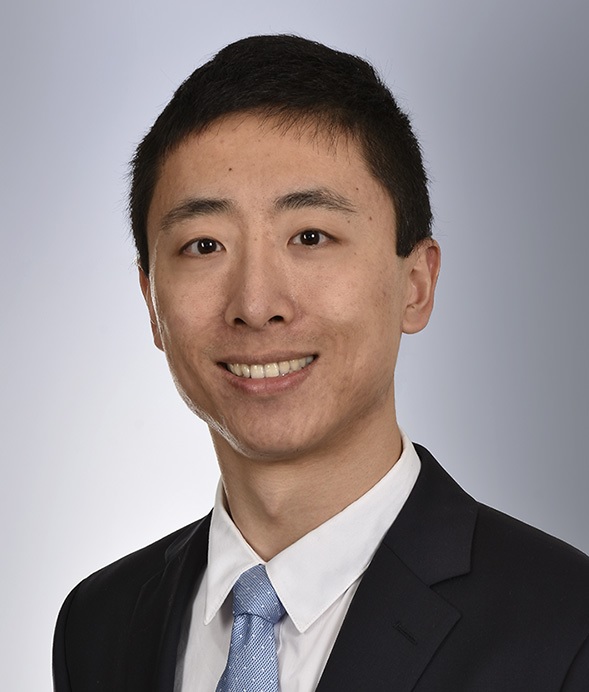
The Role of AI in Environmental Analyses
Poster Presentation
Prepared by R. Luo, T. King, O. Sosnovshchenko, C. Manwatkar, F. Avila, E. Cerda, Y. Koch
Agilent Technologies, 5301 Stevens Creek Blvd, Santa Clara, CA, 95051, United States
Contact Information: [email protected]; 470-981-6107
ABSTRACT
Per- and polyfluoroalkyl substances (PFAS), a class of emerging persistent organic pollutants (POPs), are present at trace levels not only in the environment (water, soil and air) but also in food. The quantitative analysis of PFAS is typically conducted using liquid or gas chromatography-tandem mass spectrometry (LC-MS/MS, GC-MS/MS). Despite the high sensitivity of these instruments, PFAS analysis remains challenging.
The data analysis of PFAS often requires time-consuming manual steps to eliminate false positive or negative quantifier and qualifier peaks of the corresponding compound. These steps include, among others:
• Adjusting peaks from early eluting PFAS and PFPAs (e.g. PFBA, PFOPA);
• Combining partially or fully separated peaks of linear and branched isomers of some PFAS (e.g. PFOS, PFHxS), while accounting for variations in their ratios;
• Removing false positive or negative peaks caused by matrix interferences or contamination.
In this work, we tested a complete workflow integrating several DL architectures, tailored for liquid chromatography-tandem mass spectrometry (LC-MS/MS) data in multiple reaction monitoring (MRM) mode for the quantitative analysis of PFAS. The data preprocessing workflow was designed to account for chemically relevant metadata, such as retention time shifts and quantifier-qualifier correlation. Data acquired for the analysis of PFAS in different environmental matrices following the EPA 1633 method, and from different LC-MS/MS instruments with varying sensitivities, are used for the model training and validation. Several convolutional neural network (CNN)-based architectures and a transformer-based model are evaluated and their performance compared. Preliminary results show that both models improve upon existing automatic integration algorithms. When a trained DL model is deployed, data review time can be significantly reduced by eliminating most of the manual data analysis steps, on a compound-by-compound basis.